Chunking Content: What Does Research Tell Us?
You just received the information you need to make an important decision. Which of the formats in Figure 1 appeal to you more: The left column or the right column (both have the same text)?
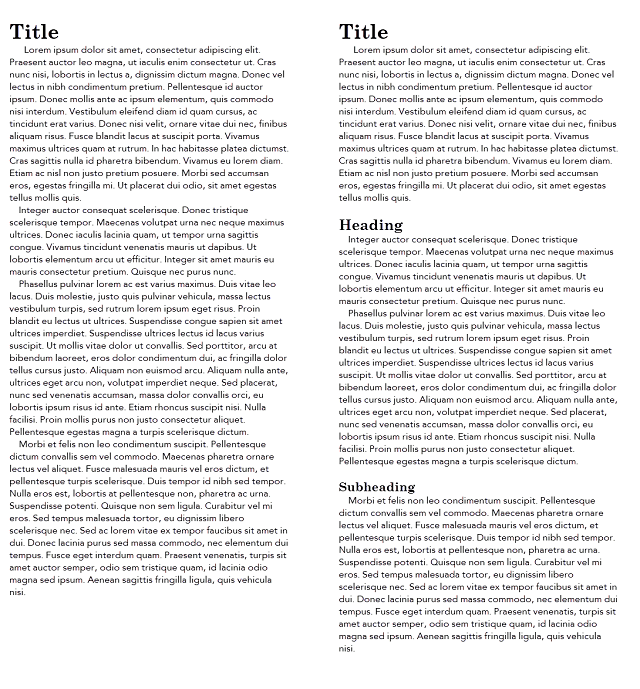
Figure 1. Undivided (left) and divided (right) version of the same content
Research by usability experts Nielsen Norman Group tells us that people prefer content that is logically divided or chunked. They define chunking as breaking up content into smaller, distinct units of information (chunks), as shown in the right column of Figure 1. This is as opposed to presenting content in homogeneous blocks like in the left column of Figure 1.
Chunking doesn’t mean simply breaking up text into smaller pieces. It means breaking them up into related, logical, meaningful, and sequential segments.
Is Chunking Content Important?
According to the Nielsen Norman Group, the reason that people prefer chunked over not chunked content is that it helps to visualize the content’s flow and organization. Chunked content doesn’t look like a wall of text, which makes content look difficult. Chunking lets people easily skim the content, which usability studies show is adults’ preferred method of reading, especially online.
Cognitive science research tells us that breaking content into logical segments makes the information easier to process, learn, and remember. Which is important for learning! This is because working memory must first process content, and it has a very limited capacity. Exceeding the limits of working memory makes it very difficult to comprehend or learn. We know that deep processing is very important to learning. Two critical goals of instruction, therefore, are to facilitate needed processing and make sure that working memory is not overloaded. I discuss and show examples of the tactics that facilitate these goals in my Deep Learning books and list a few of them below.
Facilitate needed processing | Make sure that working memory is not overloaded |
|
|
How Should We Chunk Content?
Chunking divides content in a way that makes inherent patterns and relationships clear. We can divide content according to certain features such as similarities and differences, so people can see what belongs together and what belongs elsewhere. Another common way of dividing content is by level, offering foundational information prior to more advanced and detailed information. Logically chunked content helps people more efficiently process what is presented (in working memory) and integrate new information into what is already known (in long-term memory).
What is the best way to chunk content, so people don’t think it looks difficult, it is easy to scan, and helps people learn, remember, and apply? Table 1 lists some of the major issues that should be considered and what research says.
Issue | Discussion |
Prior knowledge | We need to analyze what people already know about the topic, what they misunderstand, and what knowledge is missing. This lets us chunk according to their instructional needs. For example, if they have more prior knowledge, we can use larger chunks. If they have less prior knowledge, we should use smaller chunks. If they have misunderstandings or missing knowledge, we should include chunks that fix misunderstandings or offer missing knowledge. |
Chunk size | People cannot use prior knowledge to help them process what they are learning when they are new to a topic. Smaller chunks allow them to process a smaller amount at a time, which helps with the potential for overload. When people have more prior knowledge, we can meet their needs with larger chunks that offer targeted information for their specific needs. |
Chunk sequence | The sequence of chunks should be logical for the information offered and meet the instructional needs of the audience. For people new to a topic, for example, we often need to offer foundational information (such as terminology and underlying concepts) before more complex information. |
Chunk organization | We should organize chunks with clear headings and subheadings so that help people see how the content is organized. Levels of headings and subheadings help people literally “see” the hierarchy of topics and how they relate to each other. Content overviews (charts, maps, outlines) can reinforce content structure. |
Chunk activities | To help people deeply process the content, we should ask questions and provide relevant practice and feedback and remembering activities. We should make sure that people can fill in missing knowledge and get answers to questions, get clarification, and fix misunderstandings. |
Table 1. Chunking issues and what research advises
The Nielsen Norman Group research shows that chunking works best when we also:
- Write shorter paragraphs with white space to separate them from each other
- Highlight key words (bold or italic)
- Put lists of items into bulleted and numbered lists
- Keep related content (text and media) close together visually
Here’s the big idea: Structure information into related, logical, meaningful, and sequential segments. Each segment should contain whatever text and media goes with that segment, and each segment should be visually separate from the others.
These outcomes – motivation to understand, comprehension, retention – are important. We should chunk content according to users’ needs and design for the fact that people cannot hold more than a few information pieces in working memory at a time. George Miller researched working memory a long time ago and found that we are able to process approximately seven chunks of information at a time. That’s not a lot! More recent research suggests that seven is too high. The right number is probably three or four. The bottom line is that chunking helps us understand and remember, but it’s important to chunk logically.
References:
- Baddeley, A.D., & Hitch, G. (1974). Working memory. In G.H. Bower (Ed.), The Psychology of Learning And Motivation: Advances in Research And Theory, 8, 47–89.
- Craik, F.I.M. & Lockhart, R.S. (1972). Levels of processing: A framework for memory research. Journal of Verbal Learning and Verbal Behavior, 11, 671-684.
- Ericcson K., Chase W. G., Faloon S. (1980). Acquisition of a memory skill. Science, 208(4448), 1181–1182.
- Fountain S. B., Doyle K. E. (2012) Learning by chunking. In Seel N.M. (eds) Encyclopedia of the Sciences of Learning.
- Gilchrist, A. L., & Cowan, N. (2012). Chunking. In V. Ramachandran (Ed.), The Encyclopedia of Human Behavior (2nd ed.), 476-483.
- Gobet, F., Lane, P. C. R., Croker, S., Cheng, P. C. H., Jones, G., Oliver, I., et al. (2001). Chunking mechanisms in human learning. Trends in Cognitive Science. 5, 236–243.
- Gobet, F., Lane, P. C. R., & Lloyd-Kelly, M. (2015). Chunks, schemata, and retrieval structures: Past and current computational models. Frontiers in Psychology, 6, Article 1785.
- Hailikari, T., Katajavuori, N., & Lindblom-Ylänne, S. (2008). The relevance of prior knowledge in learning and instructional design. American Journal of Pharmaceutical Education. 72(113).
- Lah, N. C. Saat, R. M., & Hassan, R. (2014). Cognitive strategy in learning chemistry: How chunking and learning get together. Malaysian Online Journal of Educational Sciences, 2(1), 9-15.
- Miller, G. A. (1956). The magical number seven, plus or minus two: Some limits on our capacity for processing information. Psychological Review, 63(2), 81–97.
- Moran, K. (2016). How chunking helps content processing. Nielsen Norman Group.
- Sweller, J., Ayres, P. L., Kalyuga, S. & Chandler, P. A. (2003). The expertise reversal effect. Educational Psychologist, 38 (1), 23-31
- West, C., Farmer, J., & Wolff, P. (1991). Instructional design: Implications from cognitive science. Englewood Cliffs, NJ: Prentice Hall.